Abstract: Creation of a Diabetes Data Dock to Integrate, Improve, and Analyze Diverse Data Sources and Facilitate Continuous Learning and Improvement
Brent Lockee; Mitchell Barnes; Craig Vandervelden; Emily DeWit; Mark C. Clements
Children's Mercy Kansas City Kansas City, Missouri, USAbclockee@cmh.edu
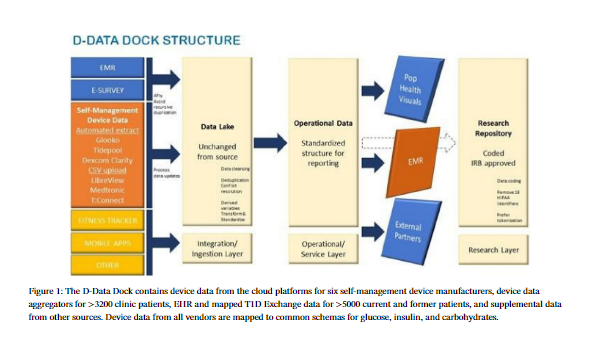
Background/Objective: Many diabetes centers within theT1D Exchange Quality Improvement Collaborative aspire to implement risk-based approaches to care, yet they face challenges mapping electronic medical records (EMR) to the T1D Exchange data standard and integrating those data with diabetes self-management device data and other sources of data to track and visualize population risk. We designed and constructed a diabetes clinic-focused cloud data infrastructure (aka, the D-Data Dock) characterized by highly reusable, general data processes that can support the diverse goals of diabetes research and clinical care.
Methods: The D-Data Dock (Figure 1) was built using Azure cloud solutions and follows a data lakehouse architecture. The design allows us to scale and adjust processes to meet the volume, variety, velocity, and veracity demands of the data.
Results: Automating the data ingestion and mapping process has enabled our team to rapidly integrate novel risk biomarkers (e.g., 6 Habits of diabetes self-management [JAMA Netw Open, 2021 Oct 1;4(10):e2131278] and CGM-based risk biomarkers [Ped Diab, 2021 Nov 22(7):982–991]) into population health dashboards and statistical process control charts to transform clinical care delivery and research. We have used the D-Data Dock to implement AI-driven predictions of near-term outcomes(e.g., hospitalization for ketoacidosis, 90-day change inA1c, 30-day change in time in range) and to pilot a remote patient monitoring program among high-risk youth.
Conclusions: The D-Data Dock is a replicable and scal-able resource ready for widespread adoption by T1DExchange member centers to improve population health management and accelerate clinical research.
Keywords: artificial intelligence, automatic, data processing, data quality, data sharing