Abstract: Predicting 90-Day Change in HBA1C with an “Explainable AI” Machine Learning Model Deployed in Clinic
C. Vandervelden1, B. Lockee1, M. Barnes1, E. Tallon2, D. Williams3, K. Noland1, R. Mcdonough1, S. Patton4, E. Dewit1, M. Clements1
1Children’s Mercy Hospital, Endocrinology, Kansas City, United States of America, 2University of Missouri, Institute for Data Science and Informatics, Columbia, United States of America, 3Children’s Mercy Hospital, Health Services and Outcomes Research, Kansas City, United States of America, 4Nemour’s Children’s Health, Center for Healthcare Delivery Science, Jacksonville, United States of America
Background and Aims: No pediatric diabetes clinics currently use advanced machine learning models to predict rise in HbA1c in youth. We developed a scalable model to predict 90-day change in HbA1c (DHbA1c).
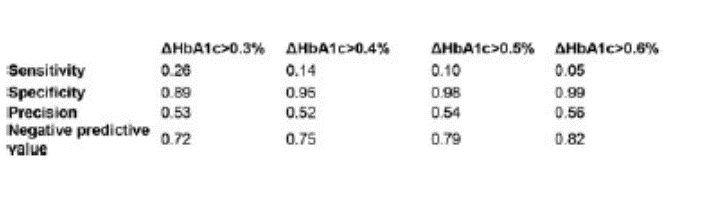
Methods: To enhance shareability, we engineered model features using electronic medical record data mapped to the T1D Exchange Quality Improvement Collaborative (T1DXQI) data schema and selected 15 features (demographics, laboratory values, and diabetes history/management) to yield explainable predictions. We used an ensemble of gradient-boosted, tree-based models and trained on data collected from 2017-09-01 to present day (2956 unique patients and 21,998 records) by a Midwestern, US diabetes center.
Results: The trained model has a root mean squared error (RMSE) of 0.71%. The most important model features are current HbA1c, T1D duration, and rate of HbA1c change. We calculated classification statistics for predicted DHbA1c>0.3% (clinically significant), 0.4%, 0.5%, and 0.6%.
Conclusions: Our DHbA1c model is an effective tool for predicting a youth’s DHbA1c 90 days after a clinic visit and is readily deployable to other centers who map their diabetes data to the T1DXQI data schema. The model enables the possibility that clinic staff can direct the highest-risk youth toward interventions (like remote patient monitoring [RPM] or behavioral interventions) to prevent HbA1c rise. Future work will add features such as device data, social determinants of health, and effective self-management behaviors.