Abstract: Predicting and Ranking DKA Risk with a Transferrable Machine Learning Model Deployed in Clinic
C. Vandervelden1, B. Lockee1, M. Barnes1, E. Tallon2, K. Noland1, D. Williams3, R. Mcdonough1, S. Patton4, E. Dewit1, M. Clements1
1Children’s Mercy Hospital, Endocrinology, Kansas City, United States of America, 2University of Missouri, Institute for Data Science and Informatics, Columbia, United States of America, 3Children’s Mercy Hospital, Health Services and Outcomes Research, Kansas City, United States of America, 4Nemour’s Children’s Health, Center for Healthcare Delivery Science, Jacksonville, United States of America
Background and Aims: No pediatric diabetes clinics have operationally deployed advanced machine learning models to predict hospitalization for DKA in youth; such models might enable development and testing of preventive interventions. We developed a scalable model to predict 6-month risk for DKA-related hospitalization.
Methods: To achieve a sharable predictive model, we engineered features using electronic medical record data mapped to the T1D Exchange Quality Improvement Collaborative (T1DXQI) data schema and chose a compact set of 15 features (demographics, laboratory values, and diabetes history/management) to yield ‘‘explainable AI’’ predictions. We used an ensemble of gradient-boosted, tree-based models and trained on data collected from 2017-09-01 to present day (2956 unique patients and 21,998 records) by a Midwestern, US diabetes center.
Results: We rank-ordered the top 10, 25, 50, and 100 (& DKA incidence, 2.5%) highest-risk youth in an out-of-sample validation set and calculated the fold-enrichment (relative to population incidence), average precision, and precision/recall curves for the 4 top-N lists (Figure).
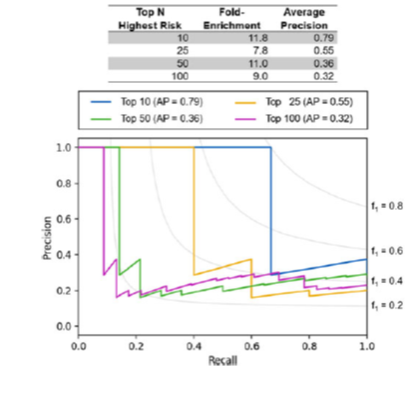
The model identified days since last DKA, cumulative DKAs, and most recent HbA1c value as the three most important features in predicting DKA risk.
Conclusions: Our DKA risk model is an effective tool for predicting a youth’s relative risk of experiencing hospitalization for DKA and is readily deployable to other centers who map their diabetes data to the T1DXQI schema. The model makes it now possible for clinic staff to contact and intervene with the highest-risk youth. Future work will add model features such as device data, social determinants of health, and effective self-management behaviors.