Abstract: Towards a Diabetes Rapid Learning Lab: Creation of a “D-Data Dock” to Integrate Diverse Data Sources and Facilitate Rapid Insights from the Deployment of Novel Interventions
B. Lockee1, C. Vandervelden1, M. Barnes1, S. Patton2, R. McDonough1, M. Clements1
1Children's Mercy Kansas City, Endocrinology, Kansas City, United States, 2Nemours Children's Health, Center for Healthcare Delivery Science, Orlando, United States
Introduction: Many diabetes centers aspire to implement risk-based approaches to care, yet they face challenges integrating electronic medical record (EMR), diabetes self-management device, and other sources of data to track and visualize population risk.
Objectives: We designed and constructed a diabetes clinic-focused cloud data infrastructure characterized by highly reusable, general data processes that can support the diverse goals of diabetes research and clinical care.
Methods: The D-Data Dock was built using Azure cloud solutions, follows a data lake house architecture, and uses a multi-hop Extract-Load-Transform (ELT) ingestion pattern. The design of the D-Data Dock allows us to scale and adjust processes to meet the volume, variety, velocity, and veracity demands of the data. Data are ingested into an Azure Data Lake while code is developed and run in a Data bricks environment. Visualizations, reports, and dashboards are published using Power BI.
Results: The D-Data Dock (Figure) now contains device data from six vendors for over 3200 clinic patients, EHR data for over 5000 current and former patients, and supplemental data from a half dozen other sources. Device data from all vendors are mapped to common schemas for glucose, insulin, and carbohydrates. Automating the data ingestion and mapping process has enabled our team to rapidly integrate novel risk biomarkers (e.g., 6 Habits of diabetes self-management [JAMA Netw Open, 2021 Oct 1;4(10): e2131278] and CGM-based risk biomarkers [Ped Diab, 2021 Nov 22(7):982–991]) to transform clinical care delivery and research. We have used the D-Data Dock to pilot an AI-informed remote patient monitoring program and begun imbedding it into EMR workflows.
Conclusions: The D-Data Dock is a replicable and scalable resource ready for widespread adoption by multiple diabetes centers to improve population health management and accelerate clinical research.
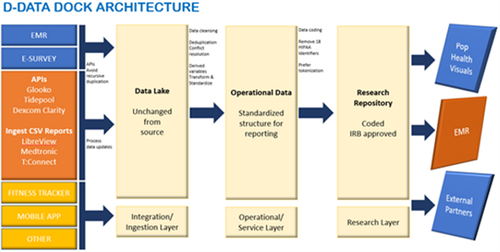